DATA+AI SECURITY SUMMIT 2024 • KEYNOTE • CHRIS ROBERTS
Smoke, Mirrors, Wings & Prayers: The Quandary of Identity, AI & Deepfakes
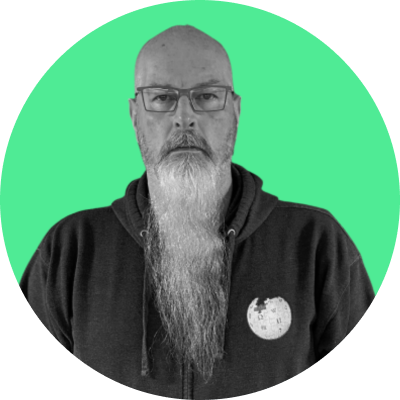
CHRIS ROBERTS
Deepfake Cyber Strategist @ WWT
All right, we’re going to actually do a briefing on both offensive and defensive. And we’ve got a nice crowd, a nice bunch of folks. So I think what we’ll do is if there’s questions, just ask them as we go along. We’ll just keep it nice and. How long am I talking for? About 30 minutes. 30 minutes. All right, we’ve got 50 slides. Hold on. We can make this work. For those of you that know me, great Those of you who don’t know me, there’s a list of crazy stuff. I like the Scottish warlock one probably more than any. Oh, and a recovering CISO as well. I did the CISO a few times. All right, so we’re going to have a conversation. As we had the As the intro basically said is, we’re going to look at both defensive and offensive, deep fake side of things here, AI, as well as some adversarial side of things. Logic here is a couple of different things. First and foremost, it’s really to understand the landscape, what it is, where it’s going and what’s happening with it. It’s also, as we probably have noticed, this thing’s accelerating faster than we can understand.
I also want to. We want to talk a little bit about the vendor landscape, who’s actually out there tackling the problems, what’s going on with it. And if it’s just a bit of a storm in a teacup, which unfortunately, it isn’t. Now, let’s have a conversation about what the hell we did to five and a half billion people on this planet. I asked AI to give me a kid holding a hand grenade and it wouldn’t give me a kid holding a hand grenade. So for those of you that are former anything we know, hand grenade equals pineapple. So I could get it to give me a pineapple and then I got it to swap a pineapple for a hand grenade, but it wouldn’t do it in the same picture. Welcome to wonderful world of AI if we take a step back, we take a step back to about November 2022. We were all going along quite happily. Everything was nice and we had AI and we had AI in what I would call a more controlled world. We had it inside DARPA. Some of us probably did the Grand Cyber Challenge. I used a bunch of my stuff in that one. We did a whole bunch of other things inside those various, various different things. But it was a controlled audience, shall we say. And then some bright spot decides to basically launch bloody ChatGPT to the entire planet.
Needless to say, five and a half billion people suddenly realized that they had all sorts of different fun things to do, which is why we ended up with this fight, flight or freeze mentality. Now some of those five and a half billion people sat there and giggled and started throwing the hand grenade at everybody else. Welcome to the adversarial side of the world. Some of them sat there and are still sitting there looking at this bloody hand grenade, wondering what the hell to do with it. Those are the people like, well, we know about AI but we don’t want to touch it. Remember the BYOD thing? Bring your own device. Hey, there’s this new thing called the telephone and people are bringing them into the offices. That’ll be fine. It’s a storm in a teacup (not) Then we had the, Then we had the other people which basically threw the hand grenade and got the hell out of there. And they’re still sitting there going, “Oh, we don’t have to touch this.” Well, too late. It’s here. And this is where we’re at from a training standpoint as we start to talk and start to deal with the human population.
If you look on basically the left side of this, we’re really good now at telling all of the people that work with us and our friends and family that know the CEO does not need any more gift cards. We are getting a little better in some circumstances at understanding some of social media and basically don’t trust the damn thing. That’s on the social media these days, but everything in the middle, that is audio and video, it’s catching us blindsided. And the irony of the whole thing is for those of us that grew up in this industry, the PBXs and everything else that we forgot about we stuffed in the corner and ignored for the last 15, 20 years because there was some new flashy shit to play with. We’ve suddenly gone, crap, we have to deal with these things again. And everything inbound is stuff that we really have to deal with. And unfortunately, the logic on us having to deal with it is because this is what we are now faced with. This is just some of the tools and some of the technology. We’ll get into this in a minute. But some of the tools and technology around the deepfake side of the world, around the ability to basically become somebody else. And as we look at this as our user population, let’s take a look at those that we are meant to protect. And I actually did some research on this. 1961, first use of passwords. 1963 or 1965, first password gets stolen. Yay. Bruce Schneier came along and said, hey, let’s put all the passwords in one place and we’ll make it nice and safe. And for a while it worked until arseholes like me came along, went, look what I can do. And then we took all the passwords and then we started to train people about password safety and password health and all this other stuff. And for the last, let’s face it, since about 1997, turn of the century, give or take a bit, we’ve tried explaining to people that 1, 2, 3, 4, 5, 6 is not a good password to use. Even adding 7, 8 and 9 on the end of it doesn’t really help very much. And yet it’s still the most popular password out there.
This is our population and we have handed to that population and we are using against that population the entirety of basically AI and deepfakes. We can’t get them to add 789 on the end of a password. We’d be screwed at this point. Even more so when we take a step back. Even more so when we take a step back and look at how easy it is to harvest credentials the old traditional way. How quickly we can get people to hand over emails, phone numbers, everything else. How quickly people fire up their computers online and hook up to the nearest network and don’t think about it. I remember 15, 20 years ago, we used to joke as people walk through DEFCON with those little earpieces and we used to end up putting them on the wall of sheep. Nowadays everybody’s at the damn stuff. We’ve kind of given up. It’s become a feature-rich attack set of vectors. And yet if you look at the bottom one, how long it takes us to educate people When you do it once a year or once a quarter for compliance, and we wonder why we’re still getting breached. Well, that’s why. So where are we today? What are we having to deal with today? This is where it gets interesting. It’s like, well, we can educate people again. So let’s take that step back. Let’s educate people. This is the list that you should be giving your users about how to stop and how to find deep fakes. That’s the list for today. Actually, that was last week’s list, to be perfectly honest, because that’s when I put this slide together. 12 things that you’re going to have to hand to your user base to explain to them how to spot a deep fake. Remember, your user base doesn’t really want to put 789 or an exclamation mark or an uppercase or a lowercase and loves clicking on shit. Is this going to work? Don’t think so. Especially when we start taking a look at all of this, how much of it is now being used across all areas of media? Obviously we’ve just gone through a rather intriguing election cycle when we had all sorts of influences from all sorts of different people. And to do that we have to take a look and see how much the explosion of AI fraud has actually impacted across the globe. This is the increases from 2022 to 2023 in percentages. 2022, everybody was going at the old fashioned way. If I’m going to take something from you, it means I have to do work.
Well, hello, good to see you too. We had to do work, we had to do all sorts of interesting stuff. 2023 comes along, I don’t have to work quite as hard. Hey, ChatGPT, give me a list of all the people that I should be breaking into. And it does, it’s very nice about it, especially if you’ve curated the data. Now if you look at it, the US a 3,000% increase. But then you start looking over at the Asia Pacific environment, an even higher increase. Why? Their adoption of technology, their ability to use technology. Think about it. The banking stuff that we now think is spectacular, they’ve had for the last five or ten years, so their adoption is higher. Unfortunately, Makes them more of a target. How much of a target? Well, same issues and challenges we have when we look at breach data. On breach longevity, we can’t agree on numbers, but we know it’s lots at the low end. FTC says 10 billion lost in 2023, 14% increase from the previous year. The Feds, bless their cotton socks, are putting at 12 and a half billion, 22% increase. AARP for those of us that have lots of gray hair and who keep getting the “Hey, join AARP,” bless their cotton socks. I think it’s like free luggage and free something else at this point in time. I get to join them. 28 and almost a half billion we’re losing. So guess what? Those adversaries out there are targeting everybody that we know and love that’s old and everybody that we know and love that’s young. So let’s take a look at where they’re focusing from a business perspective.
Three areas. Now the next couple of slides. I will apologize. I have broken all of my own personal rules, which means I put more flipping- I’ve put more flipping data on there than I need and want to. Three main areas, coverage. Coverage and speed together. Let’s face it, before we had the ability to ask an intelligence system to actually go find me data, we had to build intelligence packets. We took data, we turned it into information and we built an intelligence packet. And we were really good at it. That’s why we have an entire rooms and places filled with intelligence analysts. Nowadays, I don’t have to do that as much. I can do a one to many rather than a one to one type of attack. And I don’t care what language they speak now, because the AI stuff I use, I got 103 different languages. It speaks fairly fluently with some regional dialects, et cetera, et cetera, et cetera. The threat to organizations, poisoning of machine learning modes. This was new one. Forbes actually had something. I feel terrible standing. I can’t stand still. Forbes actually put something out. I think it was about a week or two ago when they were talking about adversaries poisoning public training modes. I’m like, well no shit, Sherlock. We’ve been doing the same thing to GitHub for years. Everybody goes out to GitHub or everybody goes out to all these places and downloads what they think is the latest and greatest bit of code to add into their project. Because let’s face it, half of the time we don’t code anymore.
We just borrow somebody else’s and we don’t think about where it Came from. Well, the same things with AI models. There are too many organizations out there that are training their AI models on public related data and not validating those models. So guess what? Let’s poison the model and then the offensive capabilities, automation. Hey, AI, give me a few different ways to get into an operating system, a company, an organization, find its faults, find its issues. We’re touting this as amazing stuff. We’re sitting there going, hey, I can have AI tell you where your faults are. Well, great, so can the adversary and they’re better at using it. Credential exploitation, et cetera, et cetera. So that’s somewhere where we’re at. So let’s take a look at the observations, which is a little smaller as well. I apologize. As it says exploit development. Why break into something when you can have an automated system do it for you? Much easier social engineering. Why should I build a campaign that I’m not sure it’s going to work? Or I build custom campaigns when I can have an automated system do it for me? Part of my job over WWT is building Deepfake videos. I’ve got a couple I’m nesting around with at the moment and we use them to demonstrate. We’re obviously asked to do it and somebody comes and says, “Hey, can you build something of my CEO telling everybody that he’s given up and he’s going off to race koalas in Tasmania?” And I’m like, absolutely. And if I’m doing a quick rush job, it’s a couple of hours and it comes up nicely and I’ve pulled any kind of public data, I’ve got their voice right and I’ve got all the harmonics right and everything else. And if I get a bit more time to play with it, I can take a bit more time. And then we post it. And then the next message from the company is like, “Don’t ever let that anywhere near the Internet.” As they do information gathering. Stealing data. It’s all about the data. And it’s ironic, we’re in somewhat of an academia environment because academia and the industries, they’re aligned to some degree but not on other degrees as it says up there. Obviously the industry is, we’re looking at it for reverse engineering and intellectual property for the most part. Whereas academia is obviously very, very concerned about the biometric spoofing, identity bypasses and everything else. We’re getting there, but for some reason or other we sit there and hide behind our Okta and our MFA and think that will protect us. Guess what? It won’t.
Threat of AI. Impersonation will become the biggest threat of all. The question becomes, how do we tell who we are? How does anybody on the other end of a digital signal really understand who the hell we are? Get into that in a little bit. So I had fun. I took the wonderful world of MITRE, which we all know and love and use and abuse, and I said, let’s talk about where we would use deepfakes, where we’d use adversarial AI. So I mapped the whole thing. Now, the fun part about this one is most people think, “Well, I don’t use it on ingress and egress,” or “I don’t use it to do engineering.” I was like, oh, hell no. And this was part of a white paper a number of us put a bunch of time and effort into, and then it got published. But the other part of this gets interesting, which is when we start doing compare and contrast with normal standard techniques across each one of those 14 areas versus building an AI model to help us. And then obviously the main line that goes up there, the nice and dark line, is the zero point. Doesn’t make a difference, obviously, as we can see, reconnaissance, a huge differentiator. And then from a Defense Evasion. Got you covered on that one. Dear AI, tell me how to avoid clown strike and all the other rubbish out there. Collection. Hey AI or hey Chat GPT. Give me everything the company has that’s of interest to ABC and D and just let it go, do what it needs to. And then, by the way, it’s going to hurt more. Yeah. So, Chris, what are the size of your orbs at the end of your lines signify?
Some of that is purely just the amount of sample groups that we were using because we got to the point where some of them was like, oh, heck, we’re in good shape on this one. And some of it was because I was trying to fit everything onto a screen and messed it up. But a big part of it from the reconnaissance stuff, that was just we had the effectiveness with the model and it’s like we didn’t need to prove it any further than we did when we started looking at the resource development. We had to look at a wider range of resource development tools and techniques. So have you tried to take this and break it down functionally and look at what the distribution is relative to the underwater candidate? Yes, and that’s a whole set of talks on itself. I’ll grab the paper. There’s a bunch of us that put a bunch of effort into it. I will find it and I’ll send it out to the team, we’ll make sure it gets out to everybody. It’s actually a, it’s a fun paper. It’s an eye opening one, should we say? So what are we doing about it? We being the, the royal we on this one, specifically on the WWT side. Well, this is where we were. I’ve been there now what, three months and change. So I passed my 90 days and everybody, you can come in please. For goodness sakes, come in, sit down, say hi, all those good things. Why am I getting a bunch of my team sitting me up and asking me if the presentations we’ve done is the same one? I’m like, oh hell no, I’ve changed it. I always change things. Anyway, when I started about three months ago, they handed this to me and they were like, hey, this is all the stuff we’ve done. I’m like, well that’s cool. That little red square down the bottom of Deepfakes and Misinformation. I said, you’re missing a few vendors. And they’re like, how many? I’m like about 70 or 80, maybe 90 or so. And they’re like, well how do you know that? I’m like, well, because here’s my spreadsheet and I have a fairly comprehensive spreadsheet and it goes across multiple pages and all this kind of good stuff.
But general background on this one, there’s about 70 odd vendors that I’m tracking that I like, dislike, don’t like have annoyed me or all those other things covering all the spectrums. As it says, audio, video, data, social media, adult extortion. That’s the nasty underlying dark belly of this industry. Here’s what’s happening. You as the CISO or you as the CEO, Most of you have families like anything. We’ve always, I wouldn’t say we’ve joked about it, but like anything, if I can’t get to you, I’ll get to your family. And I’ve always said I’ve stood up on stage and said, it’s simple. I’m going to sit there with your kid and I’m going to go. Give me your password and you won’t give me your password. So I’m like, how many fingers do you want me to break on your kid? Not nice, but welcome to how we work. Unfortunately, this is what’s happening in the AI world. They’ll take your kid and they’ll put the kid’s face or the kid’s head or anything else or the family or whatever on top of Any kind of adult material and then release it. That’s the nasty side we’re dealing with with AI. Welcome to the nasty underbelly of it.
See, there’s a whole bunch of folks dealing with that stuff. Maturity. There are a lot of AI experts out there, bless their bloody cotton socks. They’ve read three papers that think they’re a freaking expert. And most of them have decided to start companies. Some of them have got some decent ideas. There’s a whole bunch at seed stage I’m plugged into. In fact, next week when I’m out in New York, I’m sitting down with the Forgepoint boys. But I’m plugged into AS is W. We’re plugged into AS is probably a lot of this folks. We’re plugged into a lot of the seed investor. All of those folks that are out there, we’re watching a lot of those and where they’re investing money versus who’s got the good ideas, who’s likely to actually make it and who won’t. Some of them are very, very singularly focused. Most of them have gone, hey, we’re really good at audio or really good at video or other areas. Not many of them are tackling the problem at a holistic level. And we’ll talk about that in a second. Observations. As it says, most appoint solutions. Some rely on browser interactions. Kind of like the whole, hey, we can solve your problems by giving you another browser. And that doesn’t work either. Let’s face it, none of them collect everything yet.
And we’ll talk about some options that we’re building out. Most train on primarily synthetic data. This is a big nasty one. When you are talking to your vendors, get out the thumb screws. One of the most well known ones out there that spends more money on marketing than it does on research. I chewed into them pretty badly. We have them out of the clients and I can’t say who because the client wanted them. And I’m like, well, I’ll burn them down, but go for it. And we figured out they were training on about 75 to 80% synthetic data. Now if you train a machine on a machine data rather than human data and you put humans against it and you get a whole bunch of false policies, what the f*** do you expect? Seriously. So cloud based, limited on prem, et cetera, some of the issues. All right, how are we taking a look at these folks? How am I doing? Oh, shit. All right, I better speed up. I got more slides. Hold on tight. I will make sure you get these slides.
Anyway, so we’re doing a whole bunch of criteria, training, convoluted, reoccurring, generative networks, multimodal approach. In other words, what can you look at? Video, audio, et cetera, et cetera. How explainable is your AI and XAI model? So in other words, if it makes a mistake, does it realize it, does it learn from it? And near real time detection and prevention. In other words, doing this behind the scenes going, ha ha, that call that just finished. Yeah, that was a bad one. Well, no shit, Sherlock. The money just walked out of the door. So how do we get something that’s actually near real time? These are some of the folks we’re watching. Get real, love them and hate them at the same time in equal amounts. I’m actually going to have a conversation with the folks behind the scenes next week and won’t be nice. Mobbeel nice bunch of folks out of Europe that are actually doing some really nice call center stuff. They’re actually focusing a lot on the audio side of the world. Resemble AI, Imper AI. Portal, Cybermaniacs is actually awesome. If you guys haven’t messed with those folks, I love them to death. Because here’s the thing, this isn’t just a matter of solving the technology problem.
We can’t forget the human. So when you state send look at Portal 26, you’re talking a look at the business side of it. So now you can take risk, you can actually quantify it and you can put it into something the business can understand. Mimoto looks at the identity side of things. Cybermaniacs looks at the training side of it. And Reality Defender has actually got some fun tools that we’re playing with. Who else is messing around with stuff? This is just a few of the others that are starting to bubble to the top. There’s a couple. We’re not recording this, are we? We are. All right, well, there’s one that’s three quarters down that begins with P that everybody’s probably sees their tech isn’t good. We’ll just leave it at that. Why? No, I don’t care. I’ve already annoyed them. I got off a phone call with them last week, week before last, and apparently they called straight back into my bosses and complained about me. And my bosses just giggled and they said, well, if you can’t answer Chris’ hard questions, you’re screwed. So their model doesn’t hand up. It’s probably them now actual factors. So accuracy. And this is all stuff that we’re asking questions and we’re not getting the Best answers on all this kind of stuff. I’ll leave this one and we’ll fast forward, but there’s a whole bunch of stuff. We’re also asking them more things. In other words, why the hell do we even need your solution? This is this whole conversation just about AI in general. Everybody’s like, “Oh, we need AI.” Why? Not “Why do you need it?” Because it’s shiny and cool. But why the hell does the business actually need it? What is the return on investment? What are we expecting, et cetera, et cetera. Coverage. Training. Data. This is a rather interesting conversation. We’ll have a little bit more of in a second.
But garbage in, garbage out. Simple question. How many of you know wherever it physical assets are? Most of you be like, well I think I know where most of them are. Then you ask the question, how many of you know where your data is? All of it. And most people are like oh hell no. So you’re going to take a data source that you don’t know, train an AI model with data you’re not sure about and you wonder why it doesn’t work. Good fun. Here’s more things, other questions to ask when you start talking with vendors and suppliers out there, ask them all these questions and then come up with an analysis. Take a look at the risks, the viability, the ability to deliver, the ability to actually train, the talent to support it. Because again, it ain’t all about technical technologies about who do you have and how good are they and where are they. Never make any assumptions. The issues and the dependencies. both technical and non technical dependencies, and watch the adversarial side of it. So obviously part of the job we do is to build models to see if we can fool the models that they’re building from a human standpoint and a tech standpoint. That’s just a list of some of the ones that I use and from a WWT standpoint that we use to build attack models, defensive models, adversarial models against. So those are really used. If you’re not familiar with those, get familiar with them. They’re very useful. So can we actually work with the systems themselves? The answer is yes. Here’s how. Let’s break this problem down the old school way. It’s a signal. We’ve all had to deal with signals. We started messing with signals in the network days.
We played the game called “follow the packet.” Then we moved along a bit and we played the game called “follow the credit card” when PCI decided and we still play this game today. So let’s play the game again. Let’s actually break the signal down as a signal. Audio, video, text, whatever hits your infrastructure. Do some initial call identification data identification. Enrich that data. Find out what, when, why, where and how. Then there’s that call or conversational message comes in, monitor it. We know how to do spam ports. Guess what? You can do the same thing with audio, video, textual data, the whole bloody lot. We’re working with Oracle fairly extensively and there’s another bunch of folks out there. Oracle built this really cool device a couple of years ago and then didn’t know what the hell to do with it. So now we’re putting it to use. And then as the conversation carries on going, you can apply business logic. If all I want to do is maybe do a small transaction, you can have maybe a 90 or 95% probability that it’s me. If I’m moving, shifting money or doing other kinds of things, you better be damn sure it’s me.
So you can build business logic into this conversation and then on the back of it, learn. So as you talk with vendors and everybody else, this is the kind of conversations you need to have and this is how you break it down. This was me yakking away to somebody for a short period of time. It’s a signal, it’s different frequencies. You can break these down, you can do analytics on it. You can do near real time analytics on this kind of stuff. And for those people that are saying, hey, we’ll put watermarking on those signals, guess what? I got anti-watermarking stuff and it works. So let’s break it down. It’s all about signals, it’s all about interpretation, and it’s all about observation. And it’s also about the humans. We have the ability to tackle this. This is how I’m building some stuff inside. WWT’s got this kind of cool lab.
They’ve spent God knows how much money on it and then they let me play in it, which I’m kind of giggling like a school kid. And I’m building some fun stuff. Signal in initial analysis. Secondary tertiary learning. Learning model goes both technical and human model and then feedback loop. All kinds of good fun. And I’m rushing through these because I do not want to be late for everybody else. I’m getting as quick as I can. We’ll have conversations after. Anyway, building it. Signals win split signals interpretation emotive to motive. Standard social engineering, but now in a different format, observation. Can we build digital twins? Good question. Working on it. And then humans Obviously still part of the solution. We have an AI proving ground so we can get to play with this. This is the one slide I put in to go, hey, we have toys to play with. So can we trust something? We can trust data, potentially. But let’s have a conversation about how we trust the data. Let’s have a conversation about where do we put that data. We can no longer throw all of that data out and basically go woohoo. SaaS provider here have all of my data. Especially now that we’re building models.
Doesn’t work, shouldn’t work, should never have worked. And then let’s have a conversation about not just the regular data, but all the metadata that goes with it. How are you going to make decisions around that when we look at it? Some simple questions to ask yourselves. What do I have? Where is it? Who is using it versus who should be using it? Why? Just because you’ve been at the company for 20 years, it doesn’t mean you need to have access to all the damn data. Get your fucking hand slapped and get the heck off the data that you don’t need to have least privilege or something we should have had for years. And then are you sure that only the right people have the data? Simple conversations to have simple pieces of technology. Kind of part of the reason they invited me here as well. And then if you’re using this data for your modeling, is it immutable or has some pain in the ass like me started to mess with it on an ever increasing basis? And let’s talk about identities, because again, data identities, who is on the other end of the line? This is what we want. The right person, the right place, the right time and the right focus with the right motives. Right hand side is how we work to get there. Let’s take a look at the physical cars, the actual smart cars, the identity capabilities, the liveliness detection, all of these other things need to have a discussion point with them as you start looking and evaluating the vendor sphere out there. I got a whole bunch of stuff on this one, but at the end of the day, let’s face it, all any of us want to be is to be counted. We want to be seen as the human we are.
By the way, the top right hand side, please verify you are human. I got presented with that on a website and I’m like oh that’s cute. So I built a little tool and it presses it automatically because you know. Our job, why are we here? Let’s last couple of slides. This is what I want. We have one job in this industry, and that’s to protect. That’s it for those of us that jumped out of airplanes or did stupid shit in camouflage gear for years, that was our job to protect. We come into this industry, that should be our sole focus. How do we protect others around us? How can we provoke, prove who we actually are? And how do we actually have trust back, as it should be, not as a luxury. So this is where I look at things. A final reminder in the final slide. This is what I want. Right person in the right place, the right time, focus and motives. That’s all I want. As you are evaluating the vendors, the landscape, the conversations, the discussions, the data, the integrity, that is what you should be measuring against. If it doesn’t move the needle towards that from an AI deepfake, any of that kind of stuff, it’s the wrong bloody solution.